Are you considering your career in data science and simultaneously intimidated by the maths requirements?
Data science is typically based on the top of math and the amount of math required to become a data scientist is to practice maths needed for data science which is less than you are thinking about it.
The big three topics in data Science
When you search on the internet for requirements of maths for data science, ultimately, three chapters of maths will consistently shows up.
These three topics are Linear Algebra, Statistics, and Calculus. However, most positions in data science requires only statistics that you should be familiar with.
Therefore, these are the three best math courses for data science given below are:
Linear Algebra
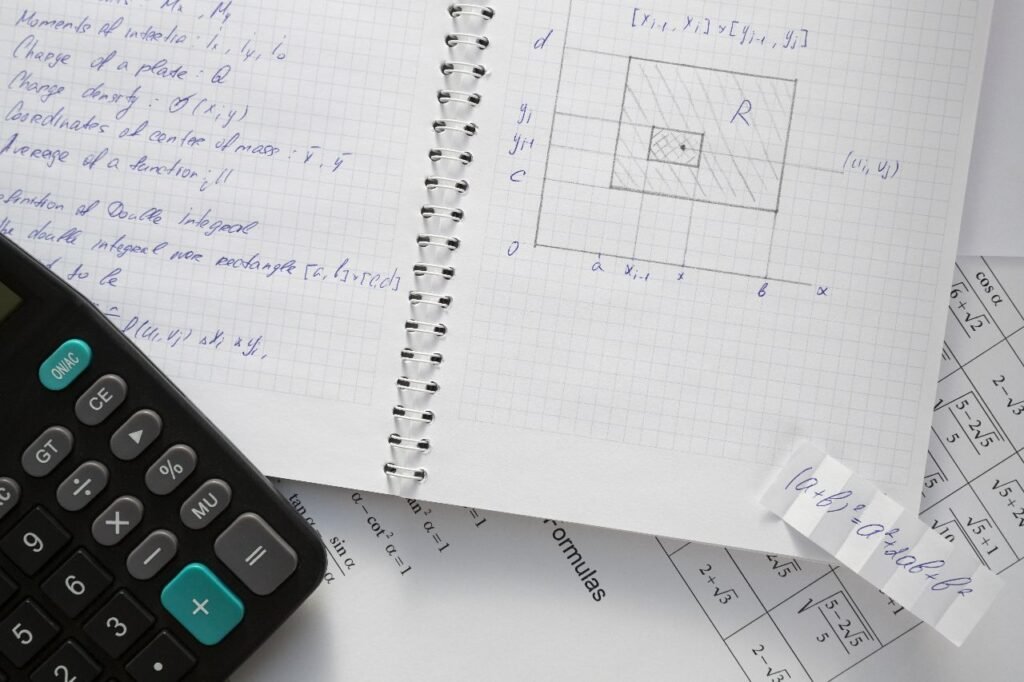
When you do data science, you will use linear algebra to perform required confirmation. If you need to perform principal component analysis for the reduction in data dimension, you will have to use linear algebra.
If you are working on neural networks, the network’s processing and representation is also going to be performed by using linear algebra. It is very hard to think about the models that do not have calculations using linear algebra.
Simultaneously, it is highly unlikely that you will have to code manually to transform the metrics while applying the models existing in a particular data set.
Therefore, understanding the principles is essential but you do not need to become a master in linear algebra maths required for data analytics to model the problem effectively.
Click Here- Unlock the Power of Data: Enroll in our Data Analytics Course Today!
Probability & Statistics
Probability and statistics is one of a kind domain where you are really going to learn something and it is the most important subject you should think about when you want to become a data scientist.
Therefore, if you do not have a strong mathematical background in probability and statistics, you need to learn to become a data scientist which is going to take time.
However, there is not a single concept that is super difficult in the data science field. You just need to take time to realize basic concepts to build your skills by practicing maths needed for data science.
Calculus
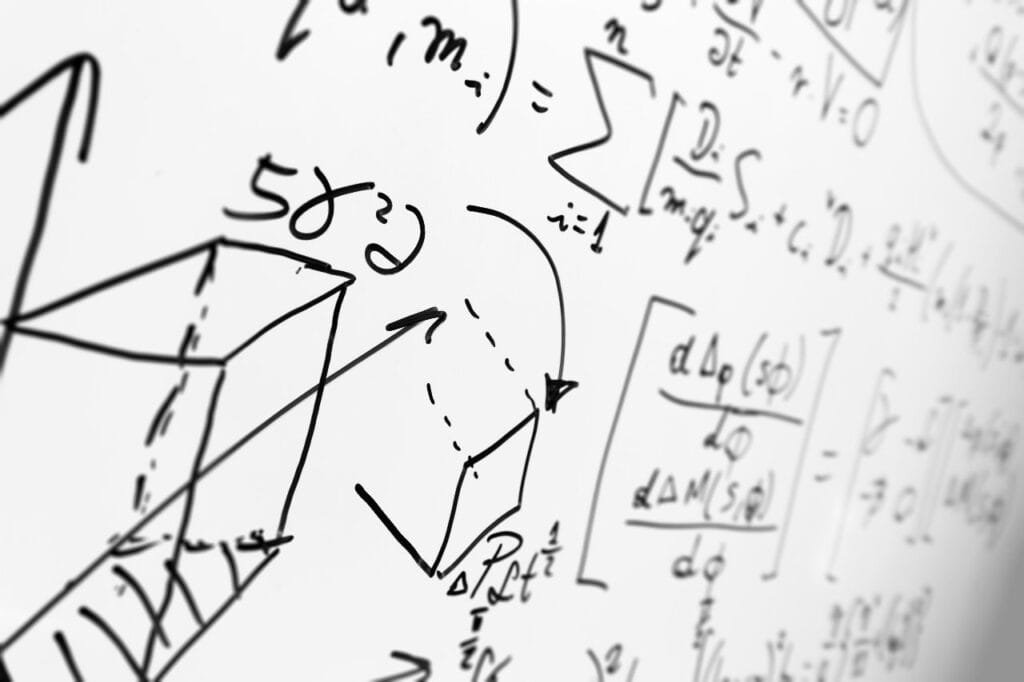
There are multiple students who have experienced traumatic experiences since high school and they thought that they would have to learn calculus. Learning calculus is one of the biggest obstacles in a student’s life to become a data scientist.
Practicing multiple data science elements depends on calculus that you may need to learn again whatever you may expect. Particularly, most data scientists understand the principles of calculus math needed for data analytics, its importance, and how it may affect data science models.
As a student, if you understand functional derivative returns the change rate. For example, if the rate of change moves towards zero then the functional trend flatters out.
In turn, calculus will help you understand the functional return and how a gradient descent works which is definitely by finding the function of local minima. It signifies that traditional gradient descent works well only for a function when they are with single minima.
Therefore, if you have multiple minima of a function, the gradient descent may find a local minima without finding the global minima unless it starts from several points.
Now, if you did your math in high school, the last few lines will blow out of your mind and sound a little dense for you. But, you can learn all the principles of maths needed for data science within an hour and it is way very less difficult for you to algebraically solve differential equations that you do not need to do at all because our computers have numerical approximations.
Click Here- Unlock the Power of Data: Enroll in our Data Analytics Course Today!
More Mathematics For Data Science And Analytics
There are many ways that may help you when you think about solving data science problems. The ways include:
Discrete Math
Discrete math is the math that deals with the finite precision of numbers. In continuous math, you may work with functions that are least theoretical but calculated for possible value sets and with necessary precision.
Simultaneously, when you start to use computers, you are in the world of discrete math in data analytics because each number has multiple “bits” available for representing the same.
Moreover, there are multiple principles from discrete math to learn for data science that serve as constraints and inspiration with the approach to solve the problems.
Graph Theory
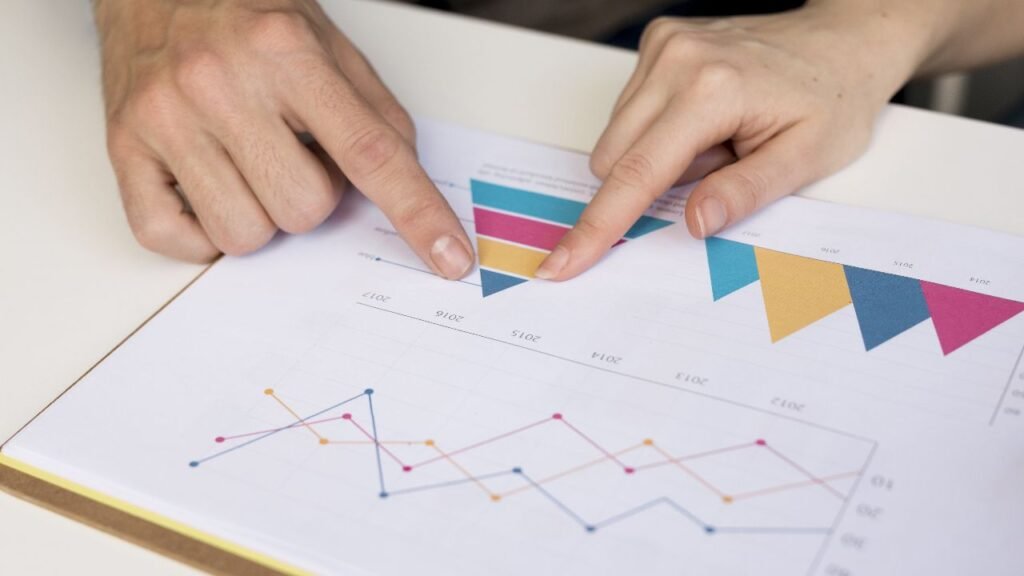
You will be able to solve certain problems by using graph theory. However, a graph-based approach will help you in optimizing routes for shipping systems or fraud detection building systems that sometimes outperform other solutions.
Information Theory
While learning data science, you are going to bump along with the information edge. Optimizing the information is of utmost important because it requires math to learn for data science.
Whether you are maximizing the retained information or optimizing it at the same time of building a decision tree, you must use Prinicipal Component analysis for data science modelling.
Click Here- Unlock the Power of Data: Enroll in our Data Analytics Course Today!
To Sum Up
In conclusion, if you are terrified or unwilling to look at the equations of maths that will help you in data science, you are not going to have fun as it is about passion.
However, if you have taken maths in your high school and willing-to-invest your time to become familiar with probability and statistics, data science is your best choice.
The maths needed for data science are probability & statistics, linear algebra, and calculus which should not be get in your way to become a professional data scientist.
Hmm is anyone else encountering problems with the pictures on this blog loading? I'm trying to find out if its a problem on my end or if it's the blog. Any responses would be greatly appreciated.
купить диплом в чите
https://qna.habr.com/user/hnfmrfdtg
купить диплом в севастополе