Statistics as used in data science, refers to the branch of mathematics that involves collecting, analyzing, interpreting, and presenting data. It encompasses a range of techniques and methodologies to extract meaningful insights from datasets and make data-driven decisions. In this blog, you would be learning about different types of important statistics for data science.
Data science involves large amounts of data to be processed, analyzed and deriving data driven decisions. Meaningful insights can be drawn from the data by identifying the patterns and trends. The unstructured data needs to be converted into a structured, numeric form which presents boundless prospects of extracting information from it. Statistics is the method to extract the information for useful insights. So, in this blog, you will explore how statistics used in data science, important statistics for data science and their uses.
What are the important statistics for data science?
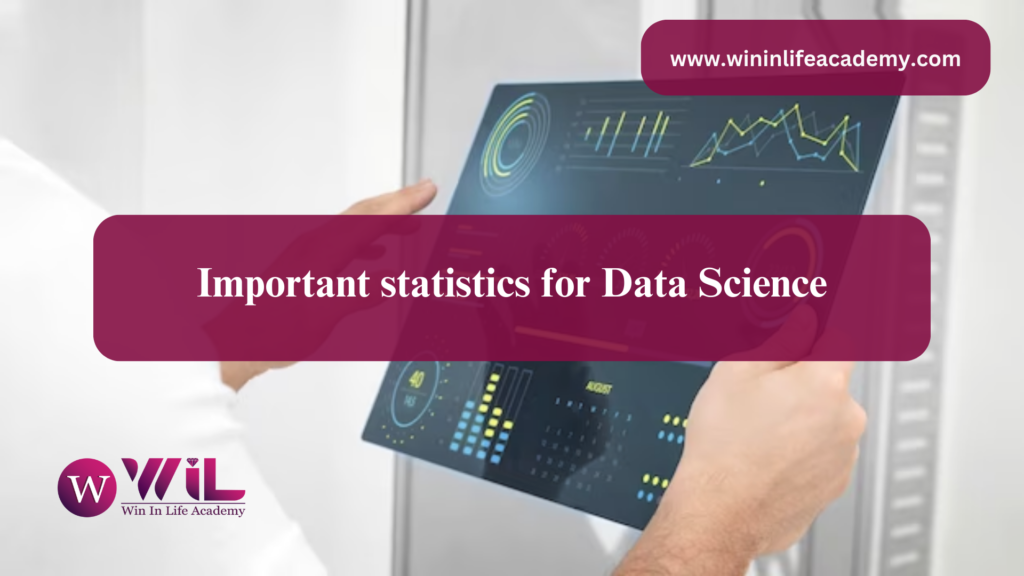
In data science, several important statistics are commonly used to gain insights and draw meaningful conclusions from data. Some of these important statistics include:
Unlock Your Career Potential. Get a Free Counselling. Enroll Now!
- Mean: Using this parameter, we can obtain the arithmetic average of values in a set. It provides a measure of central tendency and is often used to represent the typical value of a variable.
- Median: The middle value in a dataset when it is arranged in ascending or descending order. It is less affected by extreme values and provides a robust measure of central tendency.
- Mode: The most frequently occurring value(s) in a dataset. It is useful for categorical or discrete variables and can indicate the most common category or value.
- Variance: A measure of the spread or dispersion of a dataset. It quantifies the average squared difference between each data point and the mean, providing an understanding of the variability of the data.
- Standard Deviation: Square root of the variance gives standard deviation. It provides a measure of the average distance between each data point and the mean, giving an indication of how spread out the data is around the mean.
- Correlation: This statistical value specifies the strength and direction of the linear relationship between two variables. It is often represented by the correlation coefficient, which ranges from -1 to 1.
- Hypothesis Testing: A statistical method used to assess whether observed data supports or contradicts a specific hypothesis or claim about a population. It involves formulating null and alternative hypotheses and performing statistical tests to make conclusions.
- Confidence Interval: This statistical parameter gives the probability of a population parameter falling in a range of values for a certain proportion of times. It provides an estimate of the uncertainty associated with a sample statistic.
- Regression Analysis: A set of statistical processes used to estimate the relationship between a dependent variable, known as outcome, and one or more independent variables, known as predictors. It is used for predicting and understanding the impact of independent variables on the dependent variable.
- Probability Distributions: These are the mathematical functions which provide the probability of different occurrences in an experiment or event. Common distributions used in data science include the normal distribution, binomial distribution, and Poisson distribution.
The above mentioned are the best statistics for data science which form the foundation of data analysis in data science and are essential for understanding data, identifying patterns, making predictions, and drawing valid conclusions.
How statistics used in data science?
Statistics is a fundamental tool in data science, serving several crucial purposes. There is a widespread usage of statistics in data science. Statistics is a fundamental tool for collecting, evaluating, analyzing and inferring conclusions from data. Quantitative mathematical models for relevant variables are executed using important statistics for data science.
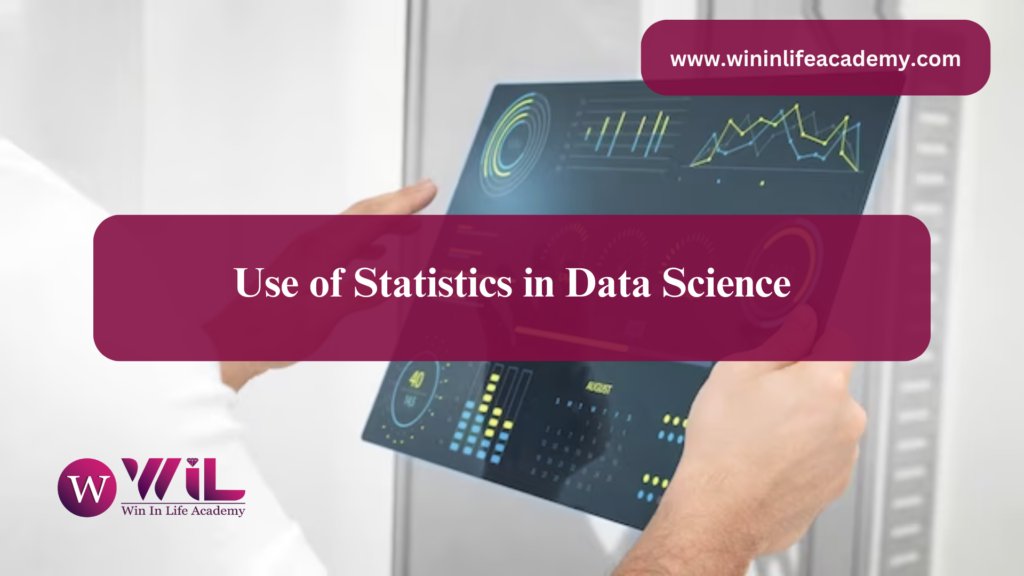
Here are some ways to see how statistics is used in data science:
- Descriptive Statistics: Data scientists employ various descriptive statistics, such as measures of central tendency (mean, median, mode) and dispersion (variance, standard deviation), to summarize and describe datasets.
- Inferential Statistics: Statistical inference is used to make predictions and draw conclusions about a larger population based on a sample of data. Techniques like hypothesis testing and confidence intervals help data scientists make statistically supported inferences.
- Probability Theory: Probability theory is a cornerstone of data science, allowing data scientists to model uncertain events and quantify their likelihood. Probability distributions, such as the normal distribution, are frequently used to describe and analyze data.
- Experimental Design: Statistics helps in designing experiments and A/B tests to assess the impact of changes and interventions. It guides the selection of sample sizes, randomization techniques, and control groups, ensuring statistically valid and reliable results.
- Regression Analysis: Regression models are employed to understand and quantify relationships between variables. These models help data scientists identify patterns, make predictions, and assess the significance of predictor variables.
- Machine Learning: Statistics provides the theoretical foundation for many machine learning algorithms. Techniques like linear regression, logistic regression, decision trees, and neural networks leverage statistical concepts to build predictive models.
- Data Exploration and Visualization: Statistical techniques facilitate exploratory data analysis, where data scientists use graphical representations and summary statistics to understand the structure and patterns within datasets.
- Anomaly Detection: Statistical methods play a crucial role in identifying outliers and anomalies in data. By comparing data points to statistical distributions or using time-series analysis, data scientists can detect unusual or suspicious observations.
Overall, you have learnt how statistics used in data science contributes to analyze, interpret, and draw meaningful insights from data, ultimately enabling informed decision-making.
How data processing make important statistics for Data Science
In the data science life cycle , a large amount of time is invested in pre-processing the data. Statistics is a powerful mechanism to remove the undesired information and organize the useful data in a straightforward way making the gigantic task of arranging inputs seem so effortless when you use best statistics for data science to analyze datasets.
Unlock Your Career Potential. Get a Free Counselling. Enroll Now!
Let us understand how important is statistics in data science.
- Data Organization: Using unstructured data can not generate any useful insights for an organization. Data needs to be classified and organized in order to work upon market plans. Statistics can help in organizing the data efficiently. If data is well ordered and categorized, it can boost the services of the company and improve the products as well.
- Identification of trends: The process of data collection can be an exhaustive process. Using statistics, it is easy for data scientists for recognizing patterns, trends in the data, thus saving huge amounts of time and cost.
- Estimation and probability distribution: Based on the real time problem, machine learning models use different types of algorithms to predict and to derive decisions. Statistics play a major role in estimation and probability distribution.
- Effective Data Visualization: Data Visualization is an essential stage in the Data Science life cycle. The large amount of complex data needs to be presented in a readable format for better interpretation of data and drawing insights. Statistical tools like histograms, pie charts, bar graphs help in analyzing the patterns and trends in the data and depiction of the results.
- Reduced dependence on assumptions: Mathematics forms the strong basis for machine learning, artificial intelligence and data analytics. Factors like differentiation and continuity help in deriving the outcomes based on precise inferences and not on assumptions. Thus, statistics enhances the strength of the model which in turn produces accurate predictions.
- Reporting variability in data: Statistics helps in reporting different variables like clusters, time, space in the data used for analysis. If statistical methods are not used, then variability might not be reported in the data analysis, which might result in incorrect estimates.
Conclusion
Statistics is a vital component of data science, providing the necessary tools and techniques to explore, analyze, and interpret data. It enables data scientists to draw meaningful insights, validate models, and make informed decisions based on statistical evidence. Descriptive statistics, inferential techniques, predictive analytics and experimental design are few of the important statistics for data science that form the backbone of data science methodologies. Its integration with machine learning algorithms enhances predictive capabilities, while decision-making under uncertainty is facilitated by statistical approaches. For aspirants who aim to grow as data scientists, it is best statistics for data science as it empowers them to extract valuable information from data, drive data-driven solutions, and shape the future of various industries.
I do not even know how I ended up here but I thought this post was great I dont know who you are but definitely youre going to a famous blogger if you arent already Cheers
Internet Chicks Great information shared.. really enjoyed reading this post thank you author for sharing this post .. appreciated
Its like you read my mind You appear to know a lot about this like you wrote the book in it or something I think that you could do with some pics to drive the message home a little bit but instead of that this is fantastic blog An excellent read I will certainly be back
helloI like your writing very so much proportion we keep up a correspondence extra approximately your post on AOL I need an expert in this space to unravel my problem May be that is you Taking a look forward to see you
Hello there! I'm glad you enjoyed my writing. I'm always happy to help out with questions or provide more information.
Connect with me at aakash@clinilaunchresearch.in
certainly like your website but you need to take a look at the spelling on quite a few of your posts Many of them are rife with spelling problems and I find it very troublesome to inform the reality nevertheless I will definitely come back again
Thank you for your feedback! I appreciate you taking the time to point out the spelling errors. It's important to me that the content I provide is accurate and professional.
I'll make sure to review and correct any spelling mistakes in my future posts.